Ethics in Data Science
- Mohd. Maaz Shaikh
- Feb 8
- 3 min read
"A man without ethics is a wild beast loosed upon this world." - Albert Camus
Ethics, is the discipline concerned with what is morally good and bad and morally right and wrong. The term is also applied to any system or theory of moral values or principles.
Ethics deals with the morality of a situation. It is the differentiating factor between making a good decision or a bad one.
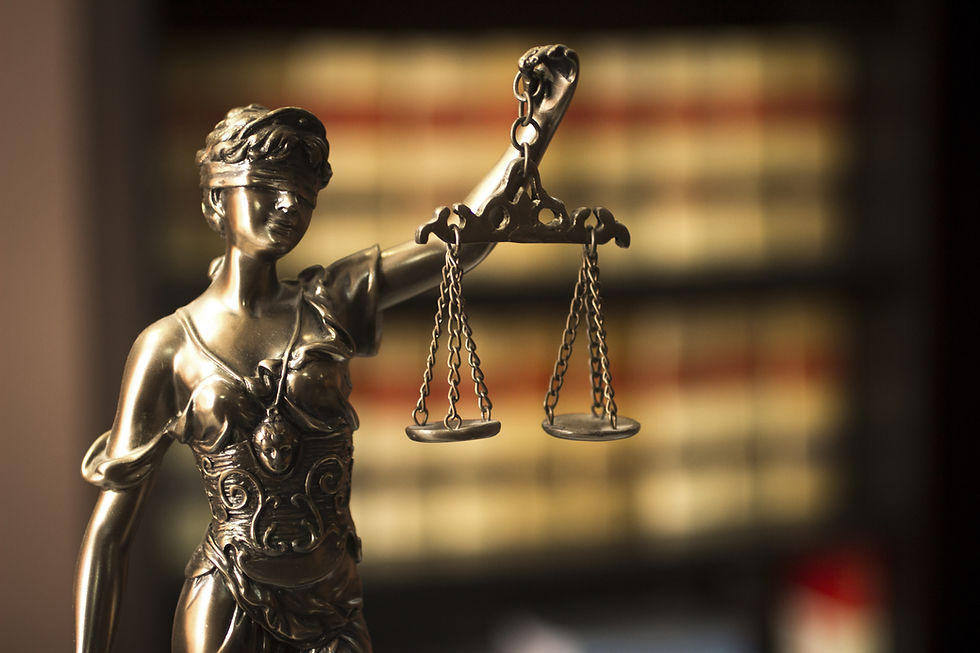
In the digital age, ethics plays a crucial role in data science, where vast amounts of personal and sensitive data are processed daily.
In todays world, data is one of the most valuable commodities. Users are generating huge amounts of data every second worldwide. This data is very valuable to many people and organizations. However people should be careful and respectful with the handling of data and make ethical choices on how to best use it.
Data Ethics is a branch of ethics which deals with evaluating the processes in data science – collecting, generating and analyzing data and how it can affect people and society. Data ethics is involved with the transparency and defensibility of data practices. Data ethics is essential in building and maintaining trust between all parties involved in the entire data science process.
A data scientist deals with vast amount of data which is sensitive in nature. These data scientists have the ability to affect the future of an organization or client by reporting their findings. Thus they have a responsibility to deal with data ethically.
Data Scientists should follow some key ethical principles:
Privacy and Protection
One of the most critical ethical considerations in data science is safeguarding individuals' privacy. Organizations must ensure that personal data is collected, stored, and processed in compliance with data protection laws. Ethical data science practices involve minimizing data collection, anonymizing sensitive information, and securing datasets from unauthorized access.
Fairness and Bias Mitigation
Algorithms are prone to bias, often reflecting historical inequalities present in training data. Unchecked bias can result in discriminatory outcomes, such as unfair hiring practices or biased lending decisions. To uphold fairness, data scientists must:
Use diverse, representative datasets.
Test models for disparate impact across different demographic groups.
Implement bias-mitigation techniques, such as re-weighting data or modifying decision thresholds.
Transparency and Accountability
Opaque decision-making processes can lead to ethical dilemmas, particularly in high-stakes applications like healthcare and criminal justice. Transparency requires making model decisions interpretable and providing explanations for automated predictions. Accountability ensures that when models fail or cause harm, responsible parties can be identified and corrective measures implemented.
Security
Cybersecurity threats pose significant risks to data integrity. Ethical data science requires implementing robust security measures, including encryption, access controls, and regular audits.
Challenges in Ethical Data Science
Despite the best intentions, enforcing ethical standards in data science can be challenging due to:
Ambiguous Regulations: Legal frameworks often lag behind technological advancements, leaving ethical gray areas unaddressed.
Trade-offs Between Accuracy and Fairness: Correcting bias in models can sometimes reduce predictive accuracy, posing a dilemma for businesses seeking optimal performance.
Corporate Pressures: Organizations may prioritize profits over ethics, leading to practices that compromise data privacy or fairness.
Ethical data science is essential for building trust, ensuring fairness, and protecting individual rights in an increasingly data-driven world. Following ethical data science practices and standards helps us navigate ethical dilemmas. Data scientists, businesses, and policymakers must collaborate to ensure that data science serves society responsibly and equitably.
Comments